Growth Insights for CEOs
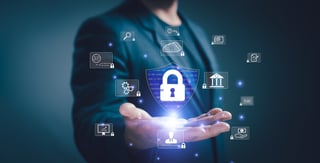
Winning the Fraud and Cybersecurity Race: A Go-to-Market Blueprint for Competitive Edge
Fraud and cybercrime have become a systemic, trillion-dollar drag on the global economy—but the fight to turn the curve is more than a market opportunity.
Over the past few years, I have worked alongside cybersecurity and fraud-management teams in government, banking, and payments, and nothing is more satisfying than seeing a new solution stop a romance scam or prevent a pensioner from losing their life savings.
Recent Posts
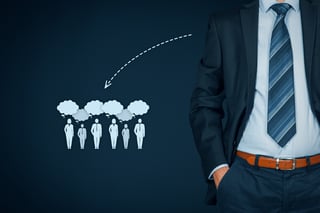
The Secret Weapon in ABM: How Research-Based Thought Leadership Transforms Prospect Relationships
Tue, Jun 3, 2025 — ABM programs that consistently outperform are those anchored in credible, research-based thought leadership. I've seen it repeatedly throughout my career as a marketing leader. In my experience, this approach leads to improved conversion from the first meeting to the next sales stages and shorter sales cycles. The secret isn't just better targeting – it's the authority established through proprietary insights.
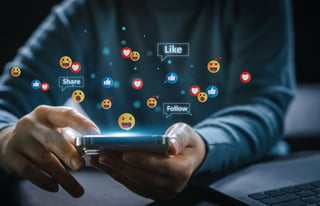
Why CEOs Must Be Active on Social Media: Building Trust and Authenticity with Customers
Wed, May 14, 2025 — I’ve seen firsthand how a CEO’s presence on social media changes how people view a company. Customers, employees, and potential hires want to know who’s steering the ship. When I show up online and share my perspective, the conversations become more genuine, and trust starts to build. It’s not about self-promotion – it’s about showing the real person behind the title.
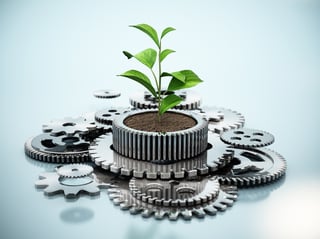
Sales Velocity Model: A Tool for Growth Engine Mechanics
Wed, May 14, 2025 — Most companies deploy a sales “force”. Too often, their combined efforts are not meeting company growth expectations. Most CEOs would like a better growth engine, a larger sales pipeline, and more sales, but they struggle with exactly how to help their sales teams. Busy CEOs combined with people and process related complexities of Sales, often results in companies not being clear on the root cause of their growth engine problems.
Stay up-to-date with the latest from Chief Outsiders
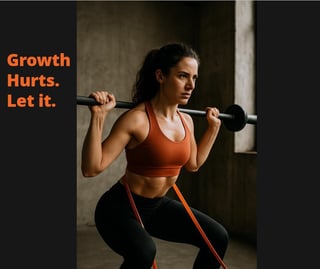
Growth Hurts. Let it.
Wed, May 14, 2025 — “Don’t Risk Too Little. Don’t Rescue Too Soon” – the growth lesson I learned outside the boardroom. My son once played striker in a school tournament. With minutes left and his team trailing, he broke through the defense and had a clean look at the goal. He hesitated, and the shot went wide.
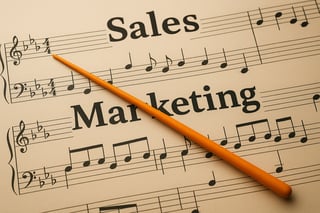
The Revenue-Ready Marketing Playbook, Part 4: How a Shared Playbook Across Sales and Marketing Unlocks Value
Fri, May 2, 2025 — This is Part 4 of the Revenue-Ready Marketing Playbook, a 4-part series for mid-market CEOs who want marketing to move the needle. You’ve got a sharp Marketing team. An experienced Sales leader. Solid product-market fit. And yet, the customer experience feels disjointed. Campaigns generate leads that go quiet after handoff. Sales calls start from scratch, even when prospects have engaged with marketing content. Messaging shifts between touchpoints and the onboarding experience doesn’t match what was sold.
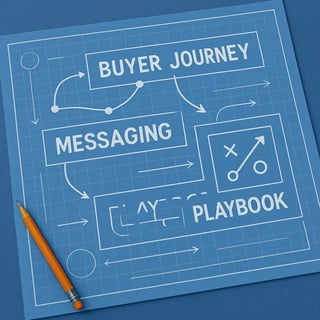
The Revenue-Ready Marketing Playbook, Part 3: How Marketing Can Deliver Predictable Outcomes
Fri, May 2, 2025 — This is Part 3 of the Revenue-Ready Marketing Playbook, a 4-part series for mid-market CEOs who want marketing to move the needle. In many boardrooms, the prevailing sentiment is "Marketing is a black box - effective in parts, but it's unclear which parts." That kind of uncertainty is surprisingly common. For many growth-stage businesses, marketing still feels like a gamble. You spend, you hope, you wonder if it worked.
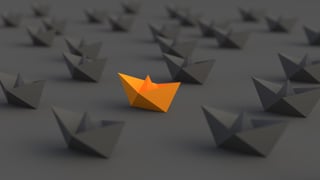
The Revenue-Ready Marketing Playbook, Part 2: In a Noisy Market, Make Clarity Your Competitive Edge
Tue, Apr 22, 2025 — This is Part 2 of the Revenue-Ready Marketing Playbook, a 4-part series for mid-market CEOs who want marketing to move the needle. A CEO once said to me, “We know our product delivers real value. Our customers are happy. But when we show up in the market, it feels like we’re saying the same thing as everyone else.” That stuck with me, not just because it was honest, but because it’s common. In crowded markets, sounding the same is the fastest way to become invisible. Buyers tune out. Sales cycles drag. Margins shrink. And despite how strong your actual offering is, you keep getting boxed in as “just another option.”
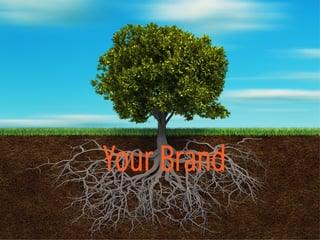
The Revenue-Ready Marketing Playbook: Why Your Brand Is Your Most Powerful Compounding Asset
Tue, Apr 22, 2025 — This is Part 1 of the Revenue-Ready Marketing Playbook, a 4-part series for mid-market CEOs who want marketing to move the needle. Each chapter explores how to turn brand, positioning, operations, and customer experience into engines for measurable growth.
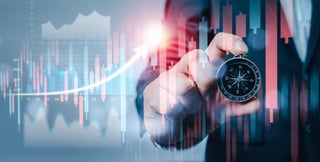
Navigating Economic Headwinds in the Consumer Products Industry
Tue, Apr 15, 2025 — Strategic Moves to Weather Uncertainty with Confidence The U.S. consumer products industry is contending with a convergence of headwinds: elevated inflation, shifting consumer priorities, and a volatile macroeconomic environment. While the instinct in challenging times may be to pause or retreat, the companies that endure and evolve are those that lean in — making strategic choices rooted in clarity, purpose, and value.